Introduction
Imagine data warehousing as the colossal bookshelf of a Harvard library, methodically organizing the world’s densest information for easy retrieval. The process of storing, sorting, and analyzing these critical business data is as integral to your company’s growth just like a perfectly brewed cup of coffee is to jumpstart your day.
Let’s get a better understanding of Data Warehousing and how it can enhance business intelligence for your business.
Understanding Data Warehousing: A Key to Better Business Intelligence
- A clear, concise definition of data warehousing.
- Why data warehousing goes hand-in-hand with business intelligence.
Definition of Data Warehousing
Data warehousing can be defined as the process of collecting, storing, and managing a vast amount of data from varied sources systematically. It’s not just a storage repository; it’s a strategic, valuable asset in the business landscape.
It’s a significant process that structures the data into one place, so it’s effectively organized, understandable, and quickly accessible. Through data warehousing, businesses have the ability to explore the historical, current, and predictive views of business operations. Hence, it serves as a foundation for decision-making – turning vast amounts of raw data into actionable business insights.
Importance of Data Warehousing in Business Intelligence
You may wonder why businesses invest in data warehousing. Primarily, it’s about gaining the edge. Businesses operate in a data-driven world where decisions are influenced by the ability to access, analyze, and understand information effectively.
1 in 2 businesses agree that data warehousing is essential in business intelligence because it helps in straightforward data analysis. It filters out irrelevant information and reduces the time spent by BI professionals on data preparation. That is – it declutters data, making it easier for businesses to comprehend trends, patterns, or insights that were previously hidden.
Data warehousing enables businesses to deliver more accurate, predictable outcomes. It helps in strategic planning by offering historical intelligence. For instance, predicting future trends or performing ‘what-if’ analyses. The result: smarter, data-driven decisions.
Finally, data warehousing improves operational efficiency. By enabling ad-hoc querying, it allows your team to find answers faster which in turn improves their productivity.
To put it simply: Data warehousing is the bridge that connects raw, unfiltered data to valuable, actionable business insights.
Exploring Data Warehouse Architecture: The Backbone of Your Data Strategy
- Understand the building blocks of data warehouse architecture.
- Learn about the different types of architecture options.
- Equip yourself with the knowledge to choose the most suitable architecture for your business.
Basic Components of Data Warehouse Architecture
A robust and functional data warehouse is not a monolithic structure but a mixture of crucial components working in sync. Stripping back to its basics, any data warehouse architecture consists of three vital parts: data sources, storage, and access tools.
Data sources are the lifeblood of any data warehouse. They can vary from operational databases, customer relationship management (CRM) systems, or even flat files. These sources feed raw data into your data warehouse.
Once inside, this information resides in a purpose-built storage system structured to accommodate and analyze large volumes of data. The storage component is designed for optimized data retrieval, ensuring your analysis isn’t held up by slow, tedious processing.
Finally, access tools provide the interface between your data warehouse and its users. Whether you’re relying on classic SQL-based tools or more modern BI platforms, these applications enable your team to extract, analyze, and report on the data stored within your warehouse, driving the crunchy insights that power your business decisions.
Different Types of Data Warehouse Architecture
Not all data warehouses are built the same. Depending on your unique business requirements, you may lean on one of the three main architecture types: centralized, decentralized, or hub and spoke. Let’s break them down:
A centralized data warehouse aggregates data from various sources into a single, unified repository. It’s a straightforward approach that generally leads to standardization and reduces data inconsistencies.
- Example: Enterprise Data Warehouse (EDW) – An EDW is a prime example of a centralized data warehouse architecture. It consolidates data from across the organization into a single repository, making it easier to report and analyze information across various departments.
- Use Case: A multinational corporation aggregates sales, marketing, finance, and HR data from different regions and departments into a centralized EDW to enable comprehensive reporting and analytics at the corporate level.
On the flip side, a decentralized architecture considers multiple smaller, autonomous data marts that serve specific departments within your organization. While it may promote agility within teams, it’s also prone to data inconsistencies due to the disconnected nature of data marts.
- Example: Departmental Data Marts – In a decentralized architecture, individual departments or business units maintain their own data marts, tailored to their specific reporting and analysis needs. These data marts operate independently of each other.
- Use Case: The marketing department of a retail company has its data mart for analyzing campaign performance, separate from the finance department’s data mart used for budgeting and financial forecasting.
The hub and spoke model integrates the strengths of both previous architectures, aggregating data into a central repository (the hub), and parsing out specialized ‘spokes’ to meet each department’s unique needs.
- Example: Hybrid Data Warehouse with a Central EDW and Departmental Data Marts – It combines elements of both centralized and decentralized architectures. The “hub” is a central data warehouse (like an EDW) that stores the organization’s integrated data, while the “spokes” are departmental data marts that contain specific subsets of data for various business units.
- Use Case: A healthcare provider uses a central EDW to store patient records, treatment data, and operational information. This EDW serves as the hub. Spokes are created for different departments, such as research, clinical operations, and billing, each with its data mart containing data relevant to their operations.
Each architecture offers its own perks and pitfalls, and discerning which one aligns with your business can be challenging.
Choosing the Right Architecture for Your Business
Choosing the best-fit data warehouse architecture isn’t a one-size-fits-all decision. It requires a deep understanding of your business needs, data strategies, and resources.
Would a centralized data warehouse streamline your workflows, or would it bottleneck your processes? Perhaps a decentralized model would foster departmental agility, but would it compromise data consistency? Would the combination of the hub and spoke model provide the best balance, or would it be too complex to maintain?
Such assessment isn’t a trivial task. It necessitates a thorough analysis of data sources, volume, business needs, and several other factors. The results of this assessment will form the blueprint for your data strategy and guide you toward the most-suited data warehouse architecture for your business.
Incorporating Data Vault for Enhanced Flexibility and Scalability
In navigating the complex landscape of data warehouse architectures, the Data Vault methodology emerges as a compelling choice for businesses looking to optimize for agility and growth. Data Vault stands out by offering a highly adaptable, scalable framework designed to accommodate the ever-evolving nature of business data.
What Sets Data Vault Apart?
Data Vault architecture employs a unique combination of Hubs, Links, and Satellites to manage data. This structure not only facilitates the efficient storage and retrieval of vast data volumes but also simplifies the integration of new data sources, making it an ideal solution for dynamic business environments.
- Hubs serve as the anchor points for business keys.
- Links connect these hubs, representing relationships between data points.
- Satellites capture the descriptive details, history, and context of the data, allowing for a rich, dimensional analysis.
The Data Vault Solution has been covered more comprehensively here.
Unveiling the Benefits of Data Warehousing: Why Your Business Needs It
- Amplified data quality and uniformity
- Boosted business intelligence
- Prolific decision-making
- Heightened operational efficiency
Improved Data Quality and Consistency
Data warehousing introduces an unmatched level of quality and consistency to your data. This is because the data comes from varied operational systems, each with its unique user input methods. When funneled into the warehouse, this data gets transformed into a unified format. This uniformity increases the accuracy, reliability, and consistency of data across the entire organization, which in turn reduces data conflicts and anomalies. In essence, data warehousing refines the “raw oil” of data into a “refined resource”, ready for savvy business usage.
Enhanced Business Intelligence
Though data alone is valuable, data warehousing facilitates further value extraction through enhanced business intelligence. This is because a warehouse houses vast volumes of historical data – offering a wealth of information about business trends and patterns. By combing through this data pool, business analysts can extract actionable insights and predict future trends more accurately. Simply, data warehousing acts as the springboard for intelligent data-driven strategies.
Better Decision Making
One direct benefit of improved data quality and enhanced business intelligence is better decision-making. When executives marshal reliable data and clear insights, they can make strategic decisions with confidence. This boosts their ability to meet and exceed business goals. Data warehousing acts as a lighthouse, providing clear, data-guided directions amidst a sea of complex business decisions.
Increased Operational Efficiency
Streamlining data access is another notable advantage of data warehousing. By consolidating data in one central repository, data warehousing abolishes the time and effort spent on retrieving data from varied sources. This allows for faster data retrieval and intensified data analysis. Moreover, because the warehouse segregates operational and analytical systems, there is reduced strain on the operational systems leading to improved performance. Data warehousing makes your business data work smarter, not harder, driving operational efficiency to a new high.
In a world where data is increasingly crowned as the ‘king’, no forward-looking business can afford to ignore the benefits of a data warehouse. Apart from the manifold advantages listed here, data warehousing offers a host of other equally significant merits. Each of these benefits significantly contributes to a corporation’s strategic advantage, fostering increased efficiency and amplified profits. The crucial role of data warehousing in sharpening business intelligence cuts through any debate – indeed, the question is not ‘if’, but ‘how’ and ‘when’ to incorporate a data warehouse.
This completes the section on the advantages of data warehousing. You now have a greater understanding of how it improves data quality and consistency, augments business intelligence, facilitates sound decision-making, and ramps up operational efficiency. These takeaways will help pinpoint the ways your business can leverage data warehousing to maximize data utilization and drive superior outcomes.
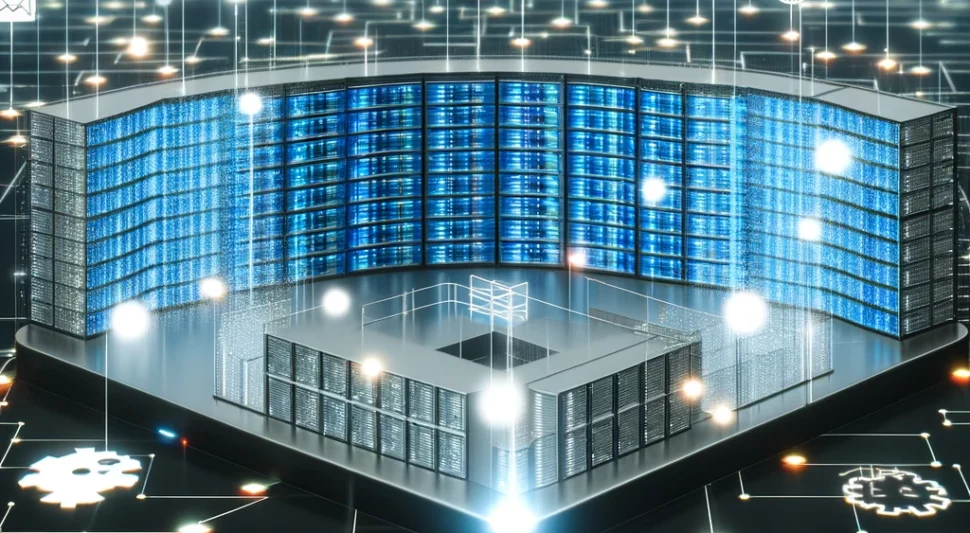
Top Data Warehouse Tools: Streamlining Your Data Management
- High-performing data warehouse designs incorporate top tools for managing, analyzing, and storing vast amounts of data.
- The right tool will vary according to specific use cases and business requirements.
- Learning from case studies can provide practical insight into successful implementations.
Data warehouses serve as the backbone of companies’ data strategies. These solutions facilitate everything from data storage and integration to analysis, and they play a vital role in business intelligence. Utilizing the optimal tools is crucial to leverage the maximum benefits from your data warehouse.
Overview of Popular Data Warehouse Tools
Data warehouses serve as the backbone of companies’ data strategies. These solutions facilitate everything from data storage and integration to analysis, and they play a vital role in business intelligence. Utilizing the optimal tools is crucial to leverage the maximum benefits from your data warehouse.
Azure SQL Data Warehouse, Amazon Redshift, Google BigQuery are just a few data warehouse tools that have made their way to the industry’s top.
Factors to Consider When Choosing a Data Warehouse Tool
Selecting an ideal data warehouse tool requires careful consideration. It can influence how efficiently your business analyzes, visualizes, and uses data. But how do you choose the right one?
- Compatibility counts. Your chosen tool should seamlessly integrate with your current tech stack.
- Scalability is another crucial factor. As your business grows, so does the data. A scalable tool will be capable of handling voluminous data gracefully.
- You also have to consider the performance, cost, security, and ease of use of the tool.
Instead of jumping on the bandwagon and adopting the tool that’s trending, businesses should make an informed choice based on their unique needs and resources. A well-chosen data warehouse tool can essentially streamline your data management, making it easier and more efficient. The right tool aligns with your business objectives, integrates with your existing architecture, and is capable of handling your data volume with ease.
Case Study: Successful Implementation of a Data Warehouse Solution
Facing the challenge of harnessing vast volumes of operational and customer data, a renowned quick-service restaurant chain with over 350 franchises worldwide sought our expertise to innovate its data management approach. In collaboration with our team, the chain selected a combination of Amazon Redshift and AWS cloud services as the cornerstone of its new data warehousing solution.
This strategic decision was driven by the need for a robust, scalable platform capable of integrating disparate data sources to provide comprehensive insights into customer preferences, sales trends, and operational efficiencies.
Implementation and Outcomes
Enhanced Data Analysis: Our implementation of Amazon Redshift enabled the team to aggregate and analyze data at an unprecedented scale, uncovering valuable insights that were previously obscured by data silos.
Customer Experience Transformation: The insights gained from the data warehouse directly informed customer engagement strategies, leading to a more personalized and satisfying dining experience. This strategic shift not only enhanced customer satisfaction but also significantly increased customer loyalty.
Operational Efficiency: The AWS cloud-based infrastructure ensured seamless data flow between the franchises, enabling real-time operational adjustments and strategic decision-making. This agility was instrumental in optimizing the chain’s operational processes, reducing costs, and improving service delivery.
Financial Growth: The cumulative effect of improved customer loyalty, operational efficiencies, and strategic decision-making fueled by data-driven insights resulted in substantial revenue growth and increased market competitiveness.
For a deeper dive into this transformation, read the full case study here.
Mastering Data Warehousing Techniques: Optimizing Your Data Strategy
- Unlock greater business insights through proficient data practices
- Boost efficiency in business operations by optimizing data strategies
- Gain a competitive edge with innovative data warehousing techniques
Data Cleansing
Data cleansing is no mere jargon; it’s a crucial element in your data warehousing strategy. This is all about sanitizing your data, reducing inaccuracies, and rectifying inconsistencies that could lead to misguided decisions. It’s like giving your data a thorough spring cleaning to ensure they are at their optimal condition.
Imagine harnessing insights from a data warehouse riddled with errors and inconsistencies – it’s akin to finding your way out of a labyrinth blindfolded. By tidying up your data, you ensure your teams can analyze data that truly reflects the business landscape.
Data Integration
Moving onto data integration. This entails blending data from different sources into a single, unified view. With this approach, you can seamlessly connect disparate data assets, making sense of them in ways that push your business forward.
For instance, data integration can facilitate the identification of patterns or trends in sales, marketing, or customer behavior. By integrating all these disparate data, you have better insights which will significantly improve decision-making.
Data Transformation
Put on your alchemist hat and transform your raw, unstructured data into meaningful information. Data transformation is like turning lead into gold: it involves converting data from its raw format into a form suitable for analytics and reporting.
Think of it as a filter that removes unnecessary details and highlights valuable insights, filtering through the data noise. By transforming your data properly, you get to grip on the insights that matter, helping your business respond to market trends swiftly.
Data Loading
Data loading is how your transformed data is loaded into the warehouse. It’s the culmination of an extensive process, transferring data from the staging area into the warehouse. But do take note – the journey doesn’t end here.
While it looks like just a simple upload, this step holds primary importance in ensuring data availability for analytic processes. The way your data is loaded into the warehouse can impact downstream analytic activities. Getting it right is akin to laying a strong foundation for your data-driven endeavor.
Throughout the journey of mastering data warehousing techniques, you’ll unravel the potentials that lie within your data. Get ready to embrace a more insightful, efficient, and competitive business horizon.
Data Warehouse vs Data Lake: Understanding the Difference
- Learn the basics of a Data Lake
- Understand the distinctions between Data Lake and Data Warehouse
- Selection guidance for Data Warehouse or Data Lake
Definition and Purpose of a Data Lake
A data lake is a vast reservoir storing massive amounts of raw data in its original form until needed. Unlike a traditional data warehouse that keeps data in files or folders, a data lake uses a flat architecture wherein each data element is assigned a unique identifier. The variety of data stored could range from structured to unstructured to semi-structured. It’s like a gigantic drawer where businesses unceremoniously stash their data — every email, every transaction, every digital interaction.
Data Lakes are instrumental in harnessing all types of data, regardless of volume or format, for scalable analytics. Their malleability makes them an excellent fit for machine learning, predictive analytics, and other data discovery or experimentation. They offer a cost-effective solution while providing potentially valuable insights from big data.
Key Differences Between a Data Warehouse and a Data Lake
While both data warehouse and data lake are highly beneficial in handling data, it’s pivotal to understand their key differences:
Feature | Data Warehouse | Data Lake |
---|---|---|
Structure | Organized, structured in tables, rows, columns, and indexes. | Raw, unprocessed, flat architecture with unique identifiers. |
Purpose and Users | Used by business professionals for decision support and BI. | Used by data scientists for analytics, machine learning, and discovering new insights. |
Data Types | Primarily structured data. | Structured, semi-structured, and unstructured data. |
Processing | Data is cleaned, transformed, and processed before storage. | Data is stored in raw form and processed at analysis time. |
Agility | Less agile but provides fast query responses. | More agile in storing diverse data types, analysis may require more effort. |
Cost | Can be expensive due to processing structured data. | Cost-effective for storing large volumes of raw data; analysis can be resource-intensive. |
Use Case | Ideal for operational reporting and BI. | Suited for big data processing, exploratory analytics, and data discovery projects. |
Choosing Between a Data Warehouse and a Data Lake
When determining whether to use a data warehouse or data lake, the nature, size, and goals of your business should serve as your guide.
If your organization depends primarily on structured data and pre-determined queries for routine reporting, a data warehouse could be the best fit. They are designed for user-friendly data exploration, offering responsiveness and performance through indexes, summarized data, partitions, and pre-calculation of complex business calculations.
However, if your organization needs to explore raw, detailed datasets to produce unique insights, data lakes could be an efficient way forward. In the data lake, users can discover new ways to use, interpret, and analyze data, making it attractive for machine learning, advanced analytics, and big data processing.
Uncertain of your next move? Consider hybrid models combining aspects of both data warehouses and data lakes. Organizations often find a multi-tier data strategy, leveraging the strengths of both systems, provides the most beneficial, flexible approach.
Remember, as your needs evolve, so too can your data strategy. It’s not a case of one being better than the other, but about finding the system that’s right for you, in your current situation. With time, you may find that your needs change, and so too must your data approach. Both data warehouses and data lakes offer valuable benefits, but their suitability depends on your specific goals and requirements. Whether you lean towards a data warehouse, a data lake, or a hybrid approach, choosing the appropriate solution can significantly enhance your business intelligence efforts.
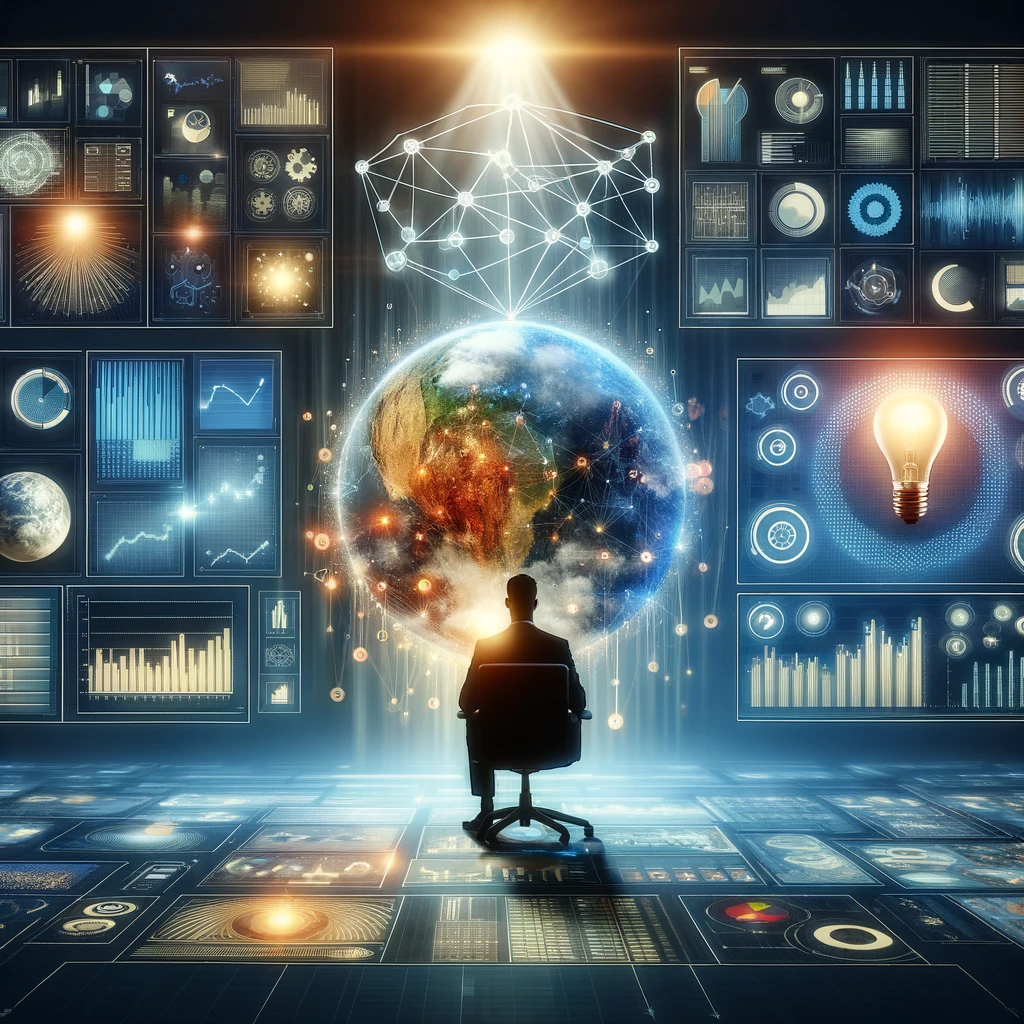
Heading into Data-Driven Progress
Data warehousing underpins effective Business Intelligence (BI), fusing diverse data pools for in-depth analysis. It lays the foundations for strategic decision-making, underpins comprehensive reporting, and propels a competitive edge – driving significant growth for your business.
Now, power up your BI with a well-designed data warehouse. Prioritize integrating previously siloed data sources, ensure quality with rigorous data cleansing mechanisms, and choose a data warehousing solution that aligns with your business goals and scales as you grow.
Ready to dive deeper into BI? Streamlining your data warehousing is your first step. Don’t rush into it recklessly – choose an experienced partner who can guide you through the process and tailor the solution to your unique needs. And remember, success in the era of big data isn’t just about having the most data, but about making the most of it. Get in touch with us today and let us guide you in the journey to a modern data warehouse.